Outdoor Autonomous Amphibious Robots
Amphibious robot can locomote in amphibious environments, including walking on rough terrains, maneuvering underwater, and passing through soft muddy or sandy substrates in the littoral area between land and water. Hence, amphibious robot is a competitive choice to replace human to execute diversified tasks in complex field environments. For example, by carrying high-performance sensors and exploration equipment, amphibious robots could independently monitor coastal environmental pollution for a long term without human intervention. To achieve autonomous performance of amphibious robots, there are several key technologies to be further studied, including the locomotion and propulsion mechanism, autonomous navigation in complex field environments and multi-robot collaboration. Autonomous navigation is the foundation of robot independent operations, however, variable illumination, rough road and dynamic objects in complex field environments will negatively affect the navigation system of amphibious robots. Multi-sensor fusion technology can make use of different sensors’ advantages and improve the system robustness. Considering limitation of a single amphibious robot, multi-robot collaboration is also important for amphibious robot to improve the mission efficient. With the continuous development of artificial intelligence, related technologies such as deep learning and reinforcement learning are expected to be applied in collaboration of amphibious robots.
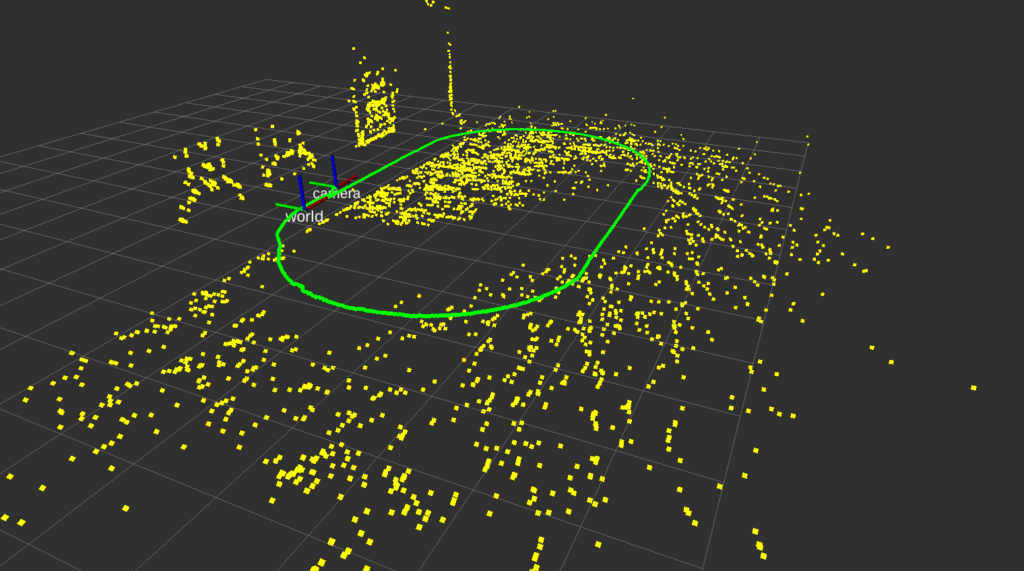
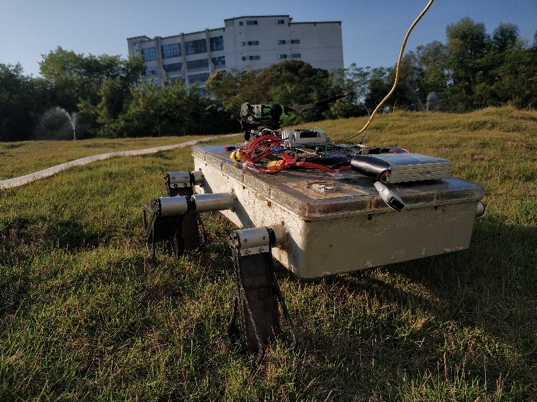
Aerial Manipulation and Transportation
Unmanned aerial vehicles (UAVs) applications are rapidly growing over the past years. In the areas of environment, construction, forestry, and agriculture, UAVs have outstanding performance especially in passive tasks such as inspection, surveillance, and monitoring. However, we still expect UAVs to conduct more active tasks, in other words, have more interaction with the environments. To improve the capability of UAVs especially in active tasks, UAV equipped with a robot arm is developed and able to interact with the world like grasping and manipulation while flying or hovering in the air.
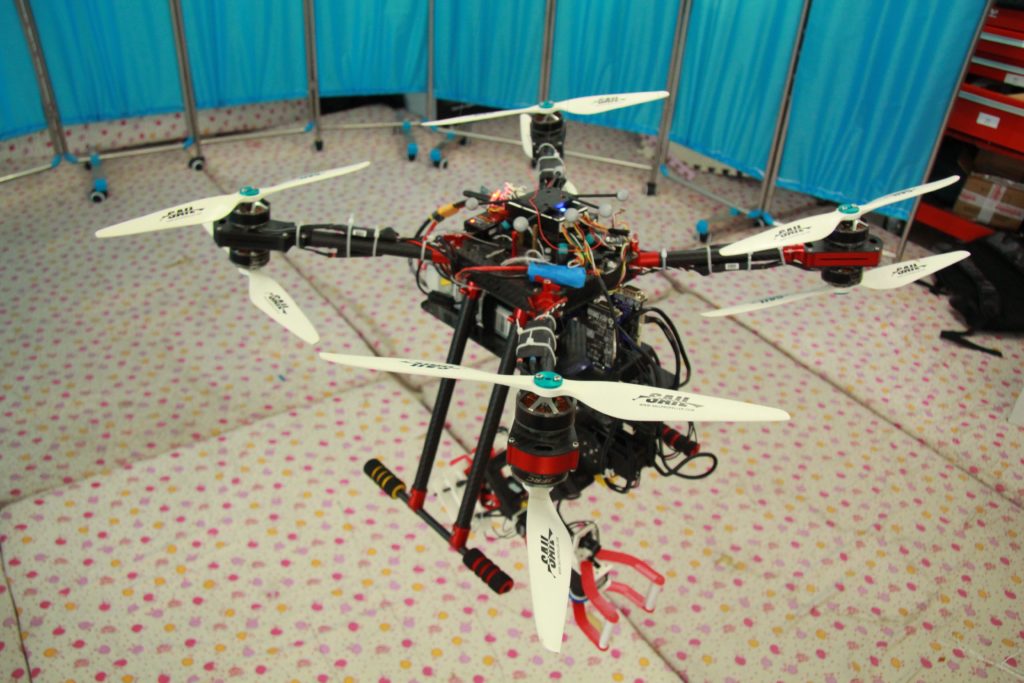
- Sensors like depth camera, fish-eye camera, IMU (Inertia Measurement Unit), and LiDAR are equipped on our aerial manipulator for its simultaneous localization and mapping (SLAM) and object detection tasks. Currently, we focus on state estimation, object recognition, active perception and large-scale mapping with semantic information in GPS-denied complex and dynamic environments.
- The planning task for our aerial manipulator includes obstacle avoidance and active exploration in unknown environments. Recent challenges include real-time obstacle-free path planning for quadrotor and motion planning for robot arm, by using low-cost computation components.
- The coupling effects between the quadrotor and the manipulator arise several control problems. We use decentralized control approach to decouple the quadrotor and robot arm and treat them separately. Our control methods include geometric control, sliding mode control, backstepping, etc.
Aerial manipulation can be used for good transportation since it can conduct pick and place tasks. It can also perform operations under dangerous or inaccessible conditions for human. Therefore, aerial manipulation has a wide application prospects in environment, agriculture, forestry, construction, search, and rescue.
Autonomous Mobile Robots in Clustered Outdoor Terrains
Simultaneous localization and mapping (SLAM) has attracted much attention because it can provide location and map information for autonomous robots in unknown environments. For example, in the field of unmanned driving and service robots, SLAM provides high-precision maps that helps robots complete path planning, autonomous exploration and other tasks. For the extremely complex operating environments, higher robustness of SLAM is required. In order to operate in the all-weather autonomous outdoor environments, unmanned vehicles need to overcome the harsh working environments such as long-term dynamic environments, strong light changing environments, highly structured urban environments, and low light environments at night.
To realize the unmanned vehicle working stably for a long time in the outdoor all-weather, we studied the key reasons for the failure of the existing vision scheme and lidar scheme in the above environments. We proposed the application scheme based on infrared camera, for which visual odometer scheme and lidar odometer scheme were fused to improve the stability of the SLAM process. At the same time, we studied the fusion positioning method based on the infrared vision and lidar sensor. By optimizing the fusion positioning framework, we finally realized the outdoor all-weather SLAM on unmanned vehicles.
Considering that the daily changes of the environments bring great challenges to the traditional slam method and have a bad impact on the effectiveness and positioning of the map, we designed a new location and mapping algorithm which combined the semantic information output from the front-end deep neural network and took the instance in the environments as the basic unit. Instead of using the traditional low-level feature methods such as descriptors or feature points, our scheme enables the robot to understand the environment more abstractly and obtain localization more robustly.
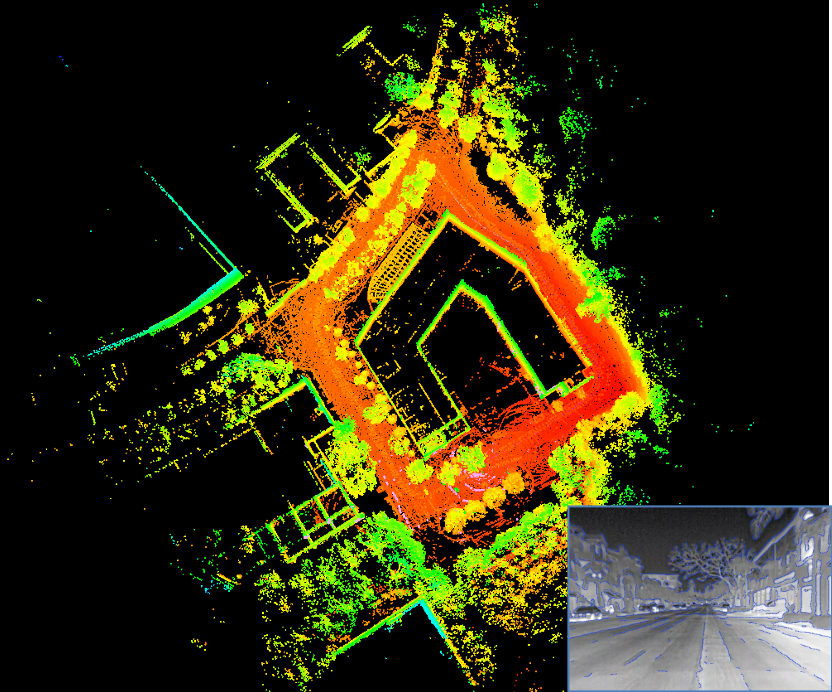
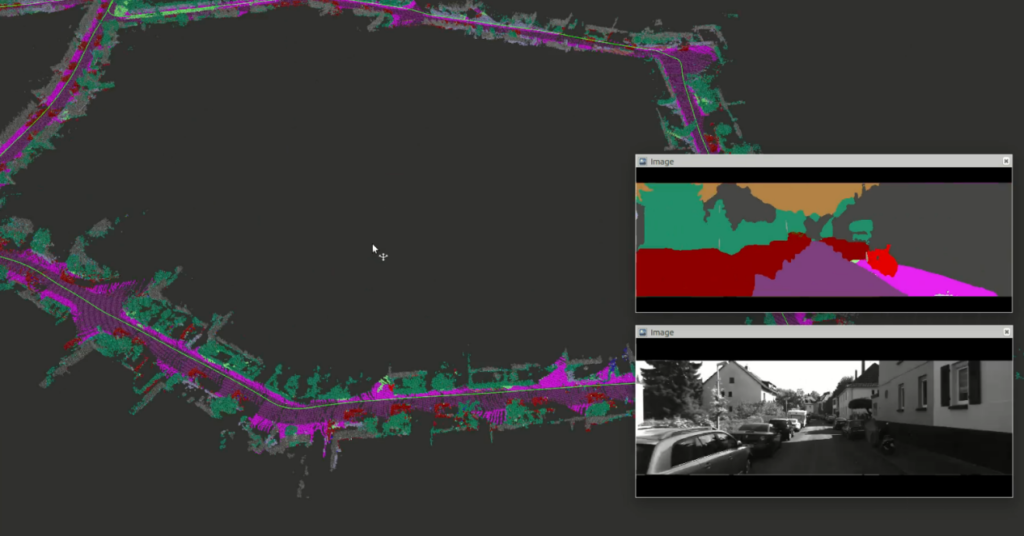
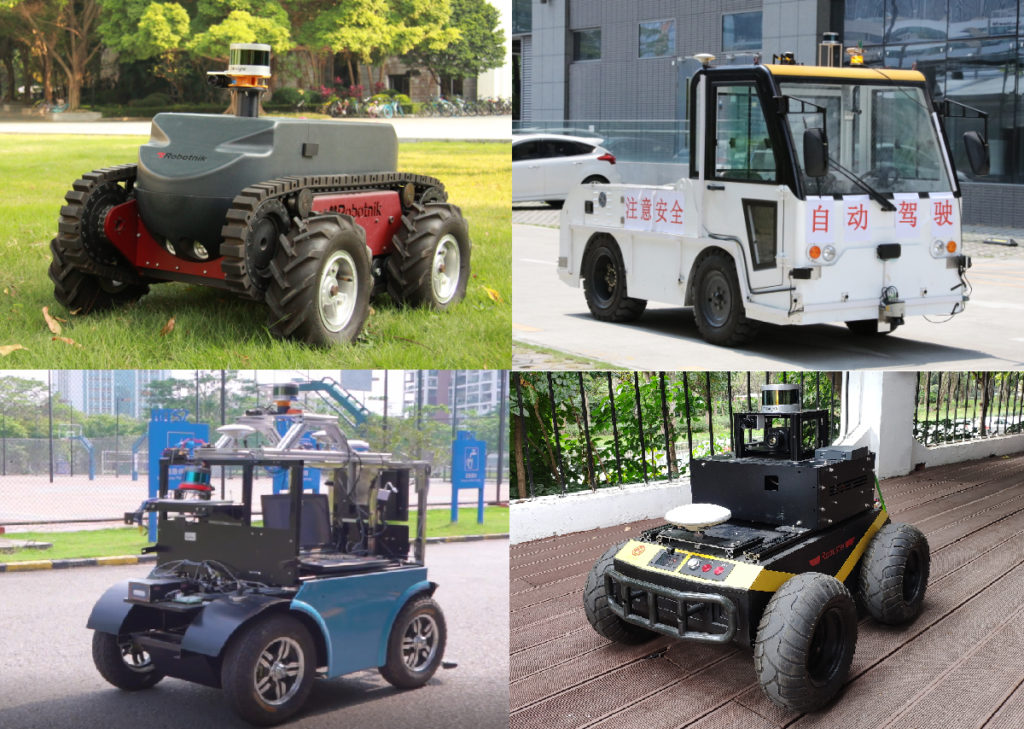
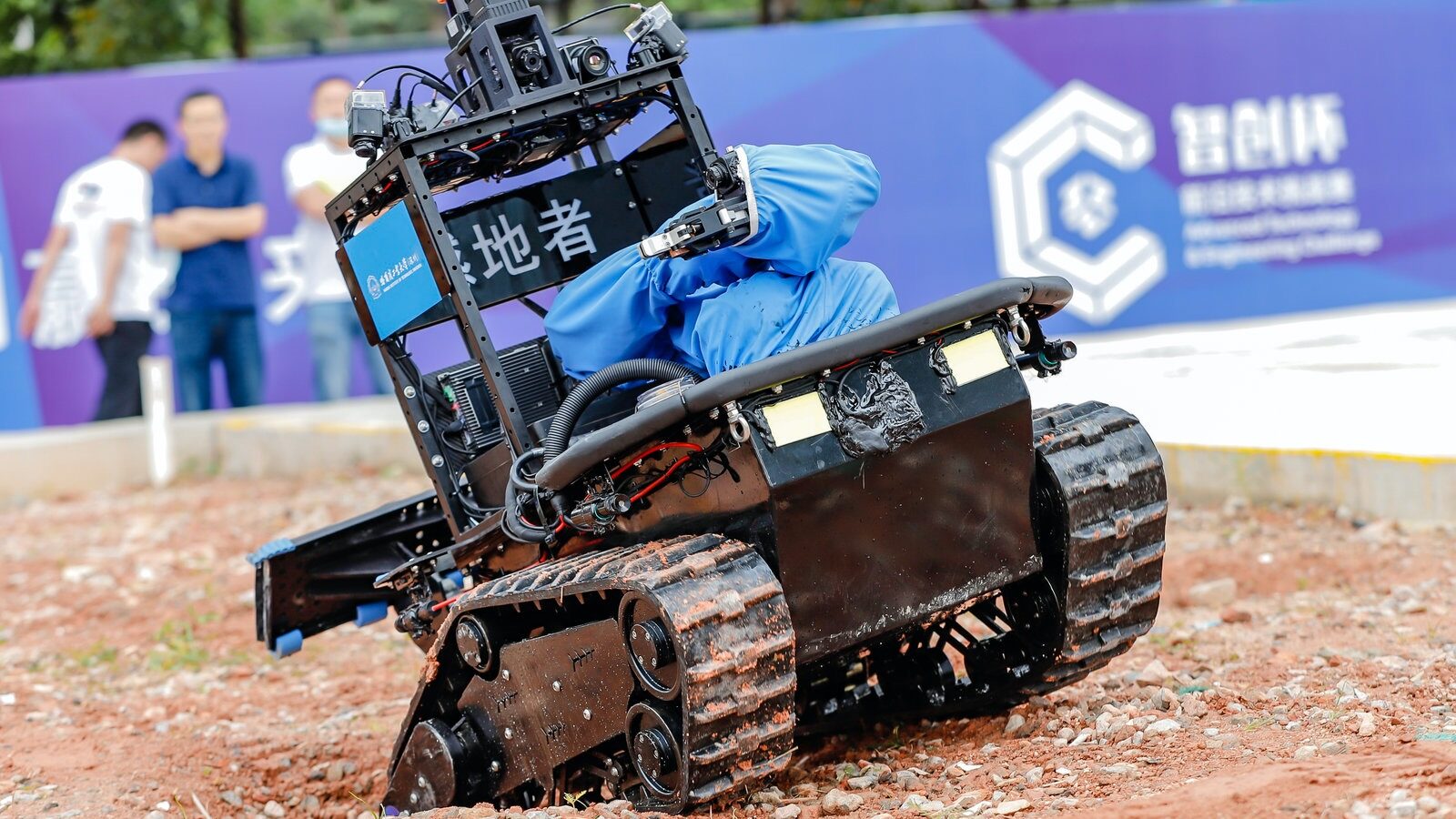
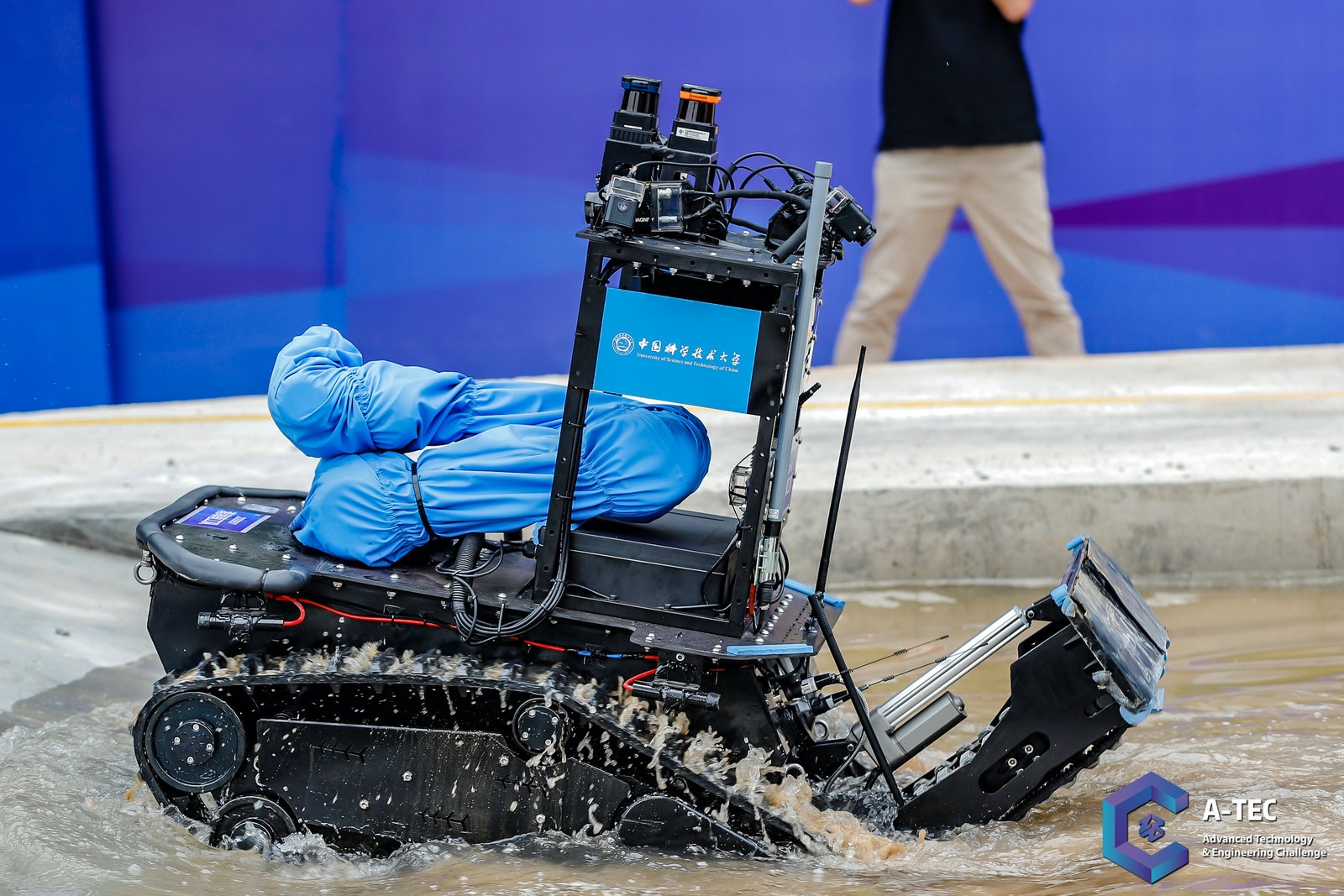